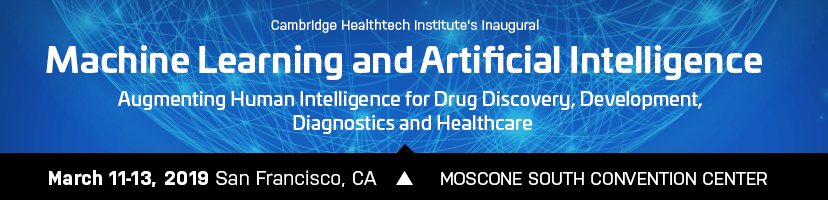
Machine learning, specifically for drug discovery, development, diagnostics and healthcare, is highly data-intensive with disparate types of data being generated that have historically been trial-and-error processes. Deep learning, machine learning (ML)
and artificial intelligence (AI), coupled with correct data, have the potential to make these processes less error-prone and increase the likelihood of success from drug discovery to the real-world setting. The Machine Learning and Artificial Intelligence
program will discuss lessons learned from case studies as well as challenges that lay ahead.
Final Agenda
Arrive Early for:
SUNDAY, MARCH 10, 2:00 - 5:00 PM (AFTERNOON SHORT COURSES)
SC6: Data Visualization - Detailed Agenda
SUNDAY, MARCH 10, 5:30 - 8:30 PM (DINNER SHORT COURSES)
SC12: Clinical Informatics: Returning Results from Big Data - Detailed Agenda
MONDAY, MARCH 11, 8:00 - 11:00 AM (MORNING SHORT COURSES)
SC23: Best Practices in Personalized and Translational Medicine
Monday, March 11
10:30 am Conference Program Registration Open (South Lobby)
11:50 Chairperson’s Opening Remarks
Joel Saltz, MD, PhD, Chair and Professor Department of Biomedical Informatics, Vice President, Clinical Informatics Stony Brook Medicine, Cherith Endowed Chair, Stony Brook University
12:00 pm NEW: Practical Applications of AI to Drug Discovery
Asim Siddiqui, PhD, CTO, NuMedii
12:30 CO-PRESENTATION: Visualizing Phenotypes from High Content Screening
Peter
Henstock, PhD, AI & Machine Learning Lead, Pfizer
Farhan Siddiqui, Advanced Analytics Architect, Pfizer
High content screening (HCS) poses a data challenge not only by the scale of the problem, but by the variety of ways that objects that can be characterized. Traditional image processing approaches have focused on identifying cells and enumerating measurements
from the images to create multidimensional feature sets. Deep learning offers opportunities to learn to recognize cell types or cellular components and quantifies them by their co-locations, counts, etc. A difficulty of both methods is providing an
interpretation of the results to the scientist. In HCS where the phenotypes are unknown, the variability between treatments and cells further complicates the ability to distinguish the classes of response and quantify how they vary from one or more
sets of controls. This talk will provide an example imaging workflow and highlight approaches for visualizing the results.
1:00 Session Break
1:10 NEW: Luncheon Presentation: Deep Learning and the Schrödinger Platform
Chris Higgs, Director, Applications Science, Schrodinger
Deep learning methods have been successfully applied to many challenging problems across diverse fields. Here we will discuss our own use of deep learning to identify hit molecules, build more predictive ADMET models, improve the efficiency of classical
scoring techniques, identify suspicious experimental data, and other preclinical drug discovery applications.
2:10 Session Break
2:30 Chairperson’s Remarks
Joel Saltz, MD, PhD, Chair and Professor Department of Biomedical Informatics, Vice President, Clinical Informatics Stony Brook Medicine, Cherith Endowed Chair, Stony Brook University
2:40 Autonomous Detection of Diabetic Retinopathy in Frontlines of Care
Ryan Amelon, PhD, R&D Director, IDx Technologies Inc.
This talk will discuss the pivotal trial of an AI system to detect diabetic retinopathy (DR) that leads to the first FDA approval of an autonomous AI diagnostic system.
3:10 Digital Pathology – Pathomics Biomarkers and Pathology Computer Aided Detection
Joel Saltz, MD,
PhD, Chair and Professor Department of Biomedical Informatics, Vice President, Clinical Informatics Stony Brook Medicine, Cherith Endowed Chair, Stony Brook University
Digital Pathology is a rapidly developing field – I will describe ongoing work to develop tools and methods that promises to improve pathology diagnostic reproducibility as well as to improve the predictive power offered by Pathology in treatment
selection and outcome prediction.
3:40 Machine Learning in Imaging
Aalpen
A. Patel, MD, FSIR, Chair, Department of Radiology, Geisinger
Deep learning has revolutionized the field of computer vision. In medical imaging, deep learning has been used in a variety of image processing tasks and recently, for diagnostic purposes such as diabetic retinopathy and skin cancer detection. Our recent
paper describes a DL-based identification of intracranial hemorrhage on head CTs and using it to prioritize the list for interpretation. Using a large clinical, heterogenous data set is very valuable in generalizing and translating to clinical tools.
4:10 How S.A.F.E. is your AI?
Vishnu Vettrivel, CTO, R&D, Wisecube
Everyone agrees that AI systems should be safe and secure throughout their operational lifetime. But in reality the safety principle is much more complex to ensure when it comes to AI. This talk will introduce a framework for safety that can help validate
whether you are using AI safely.
4:10 Building a Strong Data Foundation to Get Machine Learning Right
Reed Molbak, Product Manager, Benchling
High-quality data is a prerequisite for machine learning and advanced analytics. A first step is to ensure data is centralized and standardized. Learn how leading biopharma companies are using modern informatics to organize and structure large molecule
R&D data.
4:40 Refreshment Break and Transition to Plenary Session
8:00 Plenary Keynote Session (Room Location: 3 & 7)
6:00 Grand Opening Reception in the Exhibit Hall with Poster Viewing
7:30 Close of Day
Tuesday, March 12
7:30 am Registration Open and Morning Coffee (South Lobby)
8:00 Plenary Keynote Session Room Location: 3 & 7)
9:15 Refreshment Break in the Exhibit Hall with Poster Viewing
10:15 Chairperson’s Remarks
Alex Sherman, Director, Center for Innovation and Bioinformatics, Neurological Clinical Research Institute, Massachusetts General Hospital; Principal Associate in Neurology, Harvard Medical School
10:25 Machine Learning: From Robotics to Information Technology Products
Patryk Laurent, PhD, Director of Emerging Technology, Office of the CTO, DMGT plc
Machine learning has advanced significantly in robotics, computer vision, and the internet-of-things. Better hardware is now available, as are improved software frameworks. But industry performance requirements continually drive innovation and robustness
improvements. In computer vision, classical deep learning approaches based on pattern recognition are being challenged by neural network methods that leverage the dynamical and interactive aspects in visual signals. Illustrative examples and parallels
in non-visual domains including health care diagnostics will be discussed.
10:55 Evaluation of Algorithms from a Clinical Perspective
Sujay Kakarmath, MD, MS, Physician-Scientist, Partners Healthcare Pivot Labs
Novel predictive algorithms developed using machine learning methods are often optimized to achieve the best area under the receiver operating characteristic curve (AUC). However, this metric is often not relevant clinically. How, then, can health
professionals make conclusions about the real utility of an algorithm? The Algorithm Science team at Partners Connected Health invests a great deal of time thinking about the right questions, working out potential pitfalls and developing best
practices in evaluating machine learning-based solutions.
11:25 Generative Adversarial Networks (GAN) and Its Potential Application in Generating Synthetic Data for Clinical Trial
Shanrong
Zhao, PhD, Director, Computational Biology, Pfizer
Generative Adversarial Networks (GAN) is emerging as a disruptive method in machine learning, and has been successfully applied to text, image and voice processing. GAN has many potential applications in clinical trials as well. It can be used to
generate synthetic data and virtual patients for in silico trials, and accordingly, to reduce the cost related to the drug development and clinical trials.
11:55 NEW: Late Breaking Presentation
Alex Sherman, Director, Center for Innovation and Bioinformatics, Neurological Clinical Research Institute, Massachusetts General Hospital; Principal Associate in Neurology, Harvard Medical School
12:25 pm Session Break
12:35 Luncheon Presentation: NetApp AI Technologies Overview
Zeki Yasar, Director of
Emerging Technologies, Co-Leader AI Team, ePlus Technologies Inc.
1:05 Session Break
1:35 Refreshment Break in the Exhibit Hall with Poster Viewing
2:05 Chairperson’s Remarks
Renee Deehan Kenney, Vice President, Computational Biology, PatientsLikeMe
2:10 (How to Fix) the Very Reasonable Ineffectiveness of Machine Learning in Biomarker Discovery
Imran
Haque, PhD, Former CSO, Freenome
In this talk I will argue that our lack of complete understanding of biology and the time intensive method of sample collection have hindered biomarker discovery, and in particular that while the motivation for the empirical approach is correct, empirical
biomarker discovery has failed to grapple with data set sizes in biology that are several orders of magnitude smaller than those in other fields where machine learning has had success. Finally, I will propose lines of research to bridge the gap
between the mechanistic and empirical approaches, and in doing so address part of this data shortage.
2:40 Predicting Successful Therapeutic Targets Using Deep Learning, Matrix Factorization, and Network Propagation
Pankaj Agarwal, PhD, FRSB, Senior Fellow & Senior Director, Computational Biology, RD Target Sciences, GSK
AI and Machine Learning are being widely used in drug discovery, yet, there are significant challenges because of the lack of training examples in the biological data space. We will show three case studies examining the same problem from different
angles and using different methods. You will see the limitations of each approach and how different validation schemes impact results.
3:10 Elucidating the Determinants of Preterm Birth in the Era of Precision Medicine
Marina
Sirota, PhD, Assistant Professor, Bakar Computational Health Sciences Institute, UCSF
Survival for most children born preterm has improved considerably, but surviving children remain at increased risk for a variety of serious complications, many of which contribute to lifelong challenges. The exact mechanism of spontaneous preterm
birth is unknown, though a variety of social, environmental, and maternal factors have been implicated in its cause. In the Sirota Lab, we are in particular interested in applying computational integrative methods to investigate the role of the
immune system in pregnancy and elucidating genetic, environmental and clinical determinants of preterm birth.
3:40 To AI or Not to AI, That’s
the Question
Simon Taylor, VP ,Global Partners and Alliances, Sales, Lucidworks
Simon leads Lucidworks' search, discovery, and AI indirect channel business with resale, OEM, and integrator partners. Outside of this, Simon specializes in big data & analytics for finance, pharma and life sciences sectors aligned to the insight
engine market.
4:10 St. Patrick’s Day Celebration in the Exhibit Hall with Poster Viewing
5:00 Breakout Discussions in the Exhibit Hall
6:00 Close of Day
Wednesday, March 13
7:30 am Registration Open and Morning Coffee (South Lobby)
8:00 Plenary Keynote Session (Room Location: 3 & 7)
10:00 Refreshment Break and Poster Competition Winner Announced in the Exhibit Hall
154
10:50 Chairperson’s Remarks
Tom Defay, Senior Director, R&D Strategy and Alliances, SPMD, Strategy, Program Management and Data Sciences, Alexion
11:00 Machine Learning and Statistical Approaches for Reverse Translation
Sandor Szalma, PhD, Global Head, Computational Biology, Takeda Pharmaceuticals
We have recently implemented a global computational biology team and been expanding the capabilities to enable reverse translation across the diseases of our interest. In this presentation, I will discuss our computational infrastructural approach
and a couple of initial computational experiments to explore real-world data and machine learning methodologies to better understand patient journeys in support of the research and development organization.
11:40 Diagnosing Rare Disease Patients: Progress in Fully Automated Diagnosis
Tom Defay, Senior Director, R&D Strategy and Alliances, SPMD, Strategy, Program Management and Data Sciences, Alexion
Diagnosing patients with rare disease is challenging. Whole exome and whole genome sequencing have improved our diagnostic abilities, but in many cases many potentially disease-causing genes have potentially pathogenic mutations associated with them.
By combining phenotypic information automatically extracted from the patient’s EMR with a patient’s genome sequence, we have developed a system for proposing possible diagnoses. The effectiveness and potential utility of this approach
will be discussed.
12:30 Enjoy Lunch on Your Own
1:10 Refreshment Break in the Exhibit Hall and Last Chance for Poster Viewing
1:50 Chairperson’s Remarks
Matthew Lebo, PhD, FACMG, Director, Bioinformatics, Partners Personalized Medicine; Instructor, Pathology, Brigham and Women’s and Harvard Medical School
2:00 Machine Learning for Data Driven Decision Making of Clinical Trials
Kevin Hua,
PhD, Senior Manager, AI Machine Learning Development, Digital Health Intelligence Group, Bayer
Clinical trials are expensive business expenditures. Advances in AI/machine learning and data mining technology and availability of data make data-driven decision making possible in drug development. We would like to present a case study where wearable
devices and deep learning models are used to help clinical scientists make faster and more accurate decisions during clinical trials.
2:30 Informatics Approaches to Reducing the Sanger Burden in Clinical NGS Laboratories
Matthew Lebo, PhD, FACMG, Director, Bioinformatics, Partners Personalized Medicine; Instructor, Pathology, Brigham and Women’s and Harvard Medical School
Recent work has highlighted the accuracy and completeness of NGS such that these additional assays may not be required, especially in the realm of orthogonal confirmation of variants. However, many of these studies have been underpowered to accurately
define thresholds for ensuring high confidence in NGS variant calling. In this talk, we’ll discuss algorithmic and machine learning approaches to tackle this problem, demonstrating the ability to dramatically reduce, but crucially not eliminate,
the burden of orthogonal confirmation in germline NGS assays.
3:00 From Pixels to Phenotypes: Analysis Of Cellular Images With Multi-Scale Convolutional Neural Networks
William J. Godinez, PhD, Research Investigator, Novartis Institutes for BioMedical Research (NIBR)
Large-scale cellular imaging and phenotyping is a widely adopted strategy for understanding biological systems and chemical perturbations. Quantitative analysis of cellular images for identifying phenotypic changes is a key challenge within this strategy,
and has recently seen promising progress with approaches based on deep learning. In this talk we describe our approaches based on deep multi-scale convolutional neural networks for phenotyping cellular images. We discuss supervised as well as
unsupervised learning strategies, with the latter requiring no phenotypic labels for training. We present an example application based on images of E. Coli bacteria to show how we use machine learning to predict the binding preferences of antibiotics
directly from microscopy image data.
3:30 Session Break
3:40 Chairperson’s Remarks
Harry Glorikian, MBA, Healthcare Consultant; Author, MoneyBall Medicine: Thriving in the New Data-Driven Healthcare Market
3:45 Digitizing Human Health with Molecular and Phenome Data
Renee
Deehan Kenney, PhD, Vice President, Computational Biology, PatientsLikeMe
PatientsLikeMe has over a decade of experience collecting patient-generated health data to help individuals track information about their health and improve their outcomes. In order to leverage concomitant advances in molecular measurement technology,
we have begun collecting and analyzing biosamples on a diverse array of omics platforms, including DNA and RNA sequencing, methylomics, immunosignature, metabolomics and proteomics measurements. In this session, we will discuss the development
of a biocomputing platform that applies machine learning and other modeling techniques to aid researchers in extracting meaningful health insights from complex biological and phenomic data sets, and a case study that demonstrates the utility of
the platform.
4:15 Cancer Deep Phenotype Extraction from Electronic Medical Records (DeepPhe)
Guergana Savova, PhD, FACMI, Associate Professor, PI Natural Language Processing Lab, Boston Children’s Hospital and Harvard Medical School
We present the DeepPhe software for extracting deep phenotype information from EMRs. The software is a significant departure from other efforts in the field, as it enables comprehensive longitudinal data processing from various sources. The envisioned
applications are far-reaching, from translational clinical investigations to cancer surveillance and precision oncology initiatives.
4:45 PANEL DISCUSSION: Transformation Revolution in Laboratory Medicine
Moderator: Harry Glorikian, MBA, Healthcare Consultant; Author, MoneyBall Medicine: Thriving in the New Data-Driven Healthcare Market
Panelists: Speakers of the Session
5:15 Close of Conference Program
Stay Late for:
MARCH 14-15
S10: Data Science, Precision Medicine and Machine Learning – Detailed Agenda